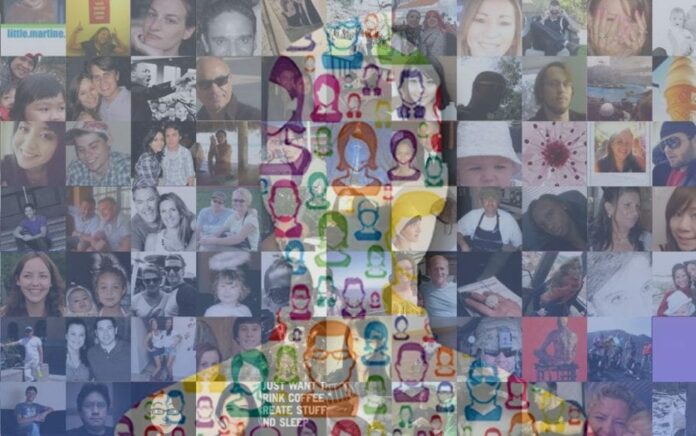
Professor Alessandro Acquisti from Carnegie Mellon University takes the floor at Black Hat USA to speak on the role of Facebook and social media in face recognition research and advancement.
Thanks everybody for being here, it’s always a great pleasure to be at Black Hat, and in this case I would like to mention my co-authors Ralph Gross and Fred Stutzman. This talk is about a few things: face recognition for sure, that’s where we start from; but face recognition is just a start. What we were interested in studying was the not so distant consequences and implications of the convergence of a number of technologies.
One of the other technologies are online social networks and, combined with a few more ingredients that you will see through my presentation, the story we are trying to tell is the story of augmented reality, of a blending of online and offline data, which I find inevitable, and which raises pretty deep and concerning privacy questions.
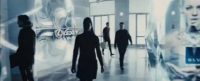
So this is what the talk is about, but I will start not from the talk itself but from the future. This is the movie that we have all seen – ‘Minority Report’. Tom Cruise is walking around a future shopping mall (see image). I believe that in the movie version of Philip K. Dick’s story, it was 2054. And somehow, the advertising is changed and adapted in real time based on the combination of technologies like face recognition and iris scan. So customers walking in the mall are recognized and advertising is targeted. The same way now online advertising is targeted based on your online behavior.
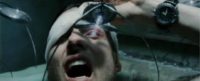
But there is also another side to this future in the movie, the creepier side, where iris scan is used for surveillance: identifying and detecting people. So keep in mind these two images, these two potential futures we are walking into. They are not mutually exclusive. Keep them in mind and we will go back to this, but in the meanwhile I will jump back into the past. I feel the best summary of the state of facial recognition today was given actually in 2010 by another Black Hat and Defcon speaker Joshua Marpet, he said: “Facial recognition sucks. But it’s getting better”. And I would strongly subscribe to these words. Computers are still way worse than humans in recognizing faces, but they keep getting better.
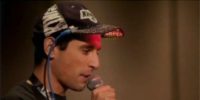
They can still be fooled. At a Defcon presentation, I believe last year or two years ago, a pretty smart idea was about putting some LEDs on a hat, which was sufficient to confuse a face recognizer (see image). This was part of the badge competition at Defcon. But they said: “Granted that face recognizer still somewhat sucks, also granted that the first derivative is clear – they keep getting better and better”.
Research in this area has been going on for more than forty years. And they keep getting better so much that they start being used in real applications – not just in security, but in fact also more recently in the Web 2.0 applications.
And in fact, if you see what has been happening in the past few months or at most a couple of years, pretty much every startup doing good work in face recognition is being acquired either by Google, or by Facebook, or by Apple. Google has acquired Neven Vision a few years ago, and then more recently Riya, and then even more recently PittPatt. PittPatt is actually interesting because it is the software that we used for our experiments. It was developed by researches at CMU (Carnegie Mellon University) where I am from. And then pretty much after our experiments were done, a few weeks ago we heard the news that PittPatt had just been acquired by Google. Similar story with Polar Rose acquired by Apple, and Facebook didn’t acquire Face.com but licensed its technology and started using it in automated tagging and so forth. So there is obviously a very significant commercial interest, as well as governmental interest in face recognition.
So what are we doing that I think is potentially different? Well, I am a researcher, I am a privacy researcher, I am not a face recognition researcher, although Ralph Gross, my co-author, is; so we took this view of extrapolating five-ten years out to what will be possible to do with face recognition: online social network, cloud computing, statistical re-identification, and data mining. So in a way, the mix, the mashup of these technologies is what can be created.
1. Continuing improvements in face recognizer’s accuracy
First of all, as I have already mentioned a couple of times, face recognition keeps improving. The FERET program, Department of Defense program to create metrics for the accuracy and performance of face recognizers, clearly shows that even just in close to ten years between 1997 and 2006, there were improvements of around two orders of magnitude.
In 1997, best face recognizer in FERET program scored error rate of 0.54
By 2006, error rate was down to 0.01
If you see the data now, although I do not know if the FERET program is still running, but if you see the performance of face recognizers now, it has gone up dramatically.
2.Increasing public self-disclosures through online social neworks
Also, something which we didn’t have that much in 2006, and definitely we didn’t have in 1997 was the incredible amount of personal information that you could find about strangers online, and in particular – photos.
In 2000 – this is the statistics I found in ‘Science’, the peer-reviewed journal – there were about 100 billion photos shot worldwide. The researchers who estimated this number did it in a very smart way: they found data on the worldwide sales of photo cameras, and then they estimated from that the number of shots that could have been made that year. Only a negligible portion of those shots made it online. But in fact, ten years later, only Facebook users, in just a month, were uploading 2.5 billion photos. Many of those photos were of course depicting faces, and many of those faces could be connected to an identity, because either they are primary profile photos or they are tagged with someone’s name. In fact, many Facebook users use their real first and last name when they create an account on the network.
3.Statistical re-identification
Other technologies that we are considering is this big mashup which creates the problem that this study is about – statistical re-identification. If I had to give a birth date to it, it would be probably 1997, when Latanya Sweeney, at the time she was at MIT (Massachusetts Institute of Technology) and then she moved on to Carnegie Mellon as a professor, discovered something that if you look back at now – it seems obvious, but truly at the time she came out with this idea, it was revolutionary.
She took people’s date of birth, which is personal information but not unique personal information. She then took people’s gender, which again is personal information but not personally identifiable information. And she took people’s zip code of the address where they live, which, once again, is personal but not unique. And combining the three, she found that 87% of America’s population were uniquely identifiable by the combination of these three pieces of data. If you imagine this as a Venn diagram1, you find out that many of us are indeed uniquely identifiable by these three pieces of data.
This is an example of the power of statistical re-identification, to start from data which is not particularly sensitive and create something which is potentially more sensitive, such as your unique identity.
The Netflix prize research by Narayanan and Shmatikov was another very good example. The scholars took data from the Netflix prize, which had been anonymized, ratings of movies, they found identity of the rater, and combined it and compared it to data from the IMDB (Internet Movie Database) and showed that they could statistically re-identify a statistically significant proportion of the members of the Netflix set.
And then of course there was our own research two years ago when we presented a study where we showed that we could predict social security numbers from public data, and in fact for instance from online social network profiles. And the story here was that we could start from something personal but not so sensitive – your date of birth and your state of birth, combine it with also publicly available data such as the Death Master File, which is a database of social security numbers of people who are dead. And by combining the two and doing some statistical analyses, we could end up predicting the SSN not of the dead people but of people who were alive.
4.Cloud computing
Another technology is cloud computing. Cloud computing allows cheaply and efficiently running many, many face comparisons in just a few seconds, otherwise that would not be possible with normal computers.
5.Ubiquitous computing
The idea of ubiquitous computing is that I can just take my smartphone and connect it to the Internet, and although my smartphone does not have the processing power to do 500 million face comparisons in seconds, something up there in the cloud can, and I just need to connect to it to run face recognition in real time in the street.
So this is what we are talking about: combining all these technologies, and in particular face recognition and publicly available online social network data, for the purpose of large-scale, automated, peer-based individual re-identification both online and offline; and individual informational inference, the inference of additional information about these individuals, potentially sensitive data.
– Face detection
– Face recognition
– Facial identification
– Facial ‘inferences’
Imagine that there are, as often in literature on statistical re-identification, two databases: an un-identified database and an identified database. In Latanya Sweeney’s example, the identified database was the voter registration list for Massachusetts voters. The un-identified database was a sensitive database of medical discharged data – obviously un-identified because hospitals wanted to share that information but they didn’t want to share it with the names of the people suffering from the different diseases. Latanya showed that you could connect the two.
So in our story the un-identified database could be images that you find on maybe match.com – a dating site; or maybe AdultFriendFinder – a dating ‘plus’ website; or prosper.com – a financial site where people look for micro funding, micro loans and often use photos because some researchers showed that profiles with photos are more likely to get funding, but they don’t use their names because they often reveal sensitive information about themselves such as their credit scores. Of course un-identified faces are also yours and mine when we walk in the street, to a stranger we are an anonymous face.
Then there are of course the identified databases, and I would argue that for most of you in this room, for most of us, there are already somewhere up in the Internet identified facial images. They may come from Facebook, if you have joined Facebook and put a photo of yourself and your real name. They could come from LinkedIn; they could come from organizations and governmental databases and so forth.
Face recognition finds a match between two images of possibly the same person, and because of this we can use identified database to give the name to a record in an un-identified database. But not only that, the story we are telling is that with the personal information that you find online, once you have a name, you can look for more information such as maybe the social security number, or maybe the sexual orientation. The paper which came out a couple of years ago showed how you could predict sexual orientation of Facebook users based on the orientation of their friends, or maybe credit scores, and because of this, if you are able to infer information for the identified database, and if you are able to find the match through recognition to the un-identified database – you close the circle, you end up connecting the sensitive data to the un-identified up till then anonymous person.
So this is the story, in a nutshell. The story is that your face is truly the veritable link between your offline persona and your online persona or personas, your many online identities. Eric Schmidt said that maybe when we turn eighteen we should be allowed to change our names to recreate from scratch our reputation. Well, but the problem is it is much harder to change your face. Your face is a constant which connects these different personas, and as I mentioned, for most of us there are already identified facial images online.
So the question is whether the combination of the technologies which are described is going to allow these linkages, linkages where it is not just that we give name to an anonymous face, but we actually blend together this online and offline data. And I want to stress this point because the SSN application that we will talk about in a few seconds is just one example, which will be shown here because we thought it would be effort to drive home the point. But it is really just one example of what is going to happen, this blending of offline and online data, this emergence of ‘personally predictable’ information that in a way is written on your face, even if you may not be aware of it.
This may democratize surveillance, and I am not saying this with a good sense, I am saying this with concern. We are not talking simply of constricted and restrained Web 2.0 applications that are limited to consenting/opt-in users, such as maybe Picasa or currently Facebook tagging. We are talking about the world where anyone in the street could in fact recognize your face and make these inferences, because the data is really already out there, it is already publicly available.
So, what will our privacy mean in this kind of future, in this augmented reality future? And have we already created a de facto ‘Real ID’ infrastructure? Although Americans are against Real IDs, we have already created one through the market place.
As I move on to describing the actual experiments that we ran to debate these questions I put here, I want to stress some of the themes I have already highlighted, because I really hope that what will remain after this talk will not be simply the numeric results of these experiments but what they imply for the future. Because I feel that what we are presenting is in a way a prototype, is a proof of concept, but five or ten years out this is really what is going to happen.
So key themes are – your face is a conduit between online and offline world, the emergence of personally predictable information, the rise of visual and facial searches where search engines allow the search for faces. Google recently started allowing pattern based searches for images, but not faces yet. Next key themes are the democratization of surveillance, social network profiles as Real IDs, and indeed the future of privacy in a world of augmented reality.
So let me talk about experiments. We did three experiments. The first one was an experiment in online-to-online re-identification. It was about taking pictures from an online database, which was anonymous or, let’s say, pseudonymous, and comparing it to an online database which was ostensibly identified – and seeing what we get from the combination of two. The second was offline-to-online: we started from an offline photo and we compared it to an online database. And the last one, the last step was to see if you can go to sensitive inferences starting from offline image.
Experiment 1: Online-to-Online Re-Identification
Experiment 2: Online-to-Offline Re-Identification
Experiment 3: Online-to-Offline Sensitive Inferences
The online database which is identified, which we have used across our experiments was Facebook profiles. Why did we use Facebook profiles? We could have used others, you know, LinkedIn profiles often, but not always, have images and names. Facebook in particular is interesting because if you read the Privacy Policy of Facebook, it really tells you that “Facebook is designed to make it easy for you to find and connect with others. For this reason, your name and profile picture do not have privacy settings”, meaning that you cannot change how visible your primary profile photo is. If you have a photo of yourself as your primary profile photo, anyone will see it, you cannot change it.
And in fact, most users do use primary profile photos which include the photos of themselves. Not only that: many users, according to our estimate – 90% use their real first and last name on Facebook profile. So you see where I am going, the story of the Real ID, de facto Real ID.
In the first experiment, we mined data from publicly available images from Facebook, and we compared it to images from one of the most popular dating sites in the U.S. The recognizer that we used for this is an application I mentioned earlier – it’s called PittPatt. It was developed at Carnegie Mellon University and has been acquired by Google recently. It does two things: first is face detection, and then face recognition. Detection is finding a face on a picture. Recognition is matching it to other faces according to some matching scores.
Identified DB – Facebook profiles
Facebook profiles were identified. Interestingly, we didn’t even log on to the Facebook to download these images. We wanted to show really that this could be done without even getting into the network. We used the API of a popular search engine to look for Facebook profiles that are likely to be in a certain geographical area. So the only thing which we could access on a Facebook profile was what is publicly available directly on the search engine, which is – for people who make themselves searchable on the search engine – your primary profile photo and your name. By the way, most users do make themselves searchable on search engines, making their profile searchable as it is also a default setting. And as we know from behavior decision research, people tend to stick to default settings.
We had the ‘noisy’ profile search pattern. So we had to write code to try to infer who these people in these geographical areas were. It used to be a few years ago that this task would have been much easier because Facebook at that time was actively using regional networks. This is no longer the case. However we have something such as Current Location which we used, plus we used another combination of searches such as whether they were members of for instance colleges in the same area, whether they were fans of companies or teams in the same area and so forth. Obviously, this is a ‘noisy’ search.
274,540 images
110,984 unique faces
Unidentified DB – Dating site profiles
The second database was the dating site, not identified because people, members of dating sites of course use photos, but also use pseudonyms. You have to use a photo if you want to go out on a date because researches show that if you don’t, the chances that someone replies to your invitation are pretty slim. However, people don’t like to put their real names, there is still an element of social stigma and therefore people use pseudonyms in online dating sites. However, because they use their facial, frontal photos they can be identified by friends who happen to be on the same dating site or perhaps by strangers.
If you had to do this comparison manually it would be unrealistic, impossible. You would have hundreds of millions of face comparisons to do. So no human being can really take the time of having one browser open on Facebook and the other browser open on the dating site, and hope to find matches. But of course this is not a problem for a computer, especially when you start using in parallel cloud computing, because it allows millions of face comparisons in seconds.
4,959 unique faces
So this is in a nutshell what we did. These are fake images by the way; I am not exposing the private data of real dating site users. So we started from a dating site image and we compared it to an image we found on Facebook – and we ended up finding the Facebook profile, and then we could give the name to the person on the dating site.
Ground truth
Now, the overlap between the two sets is obviously noisy. Why? Because our search for the Facebook profile was based on keywords, not really on geographical search. So it was a little bit noisy. We cannot know exactly the overlap between the two, and we also don’t know what the ground truth is, in a sense that we don’t know exactly how many Facebook users are also members of the dating site, and how many members of the dating site are also members of Facebook.
So before we actually ran the experiment I was describing, and I am stepping back for a second, we ran two surveys online: one with subjects, users, participants from the same geographical area I am talking about; and one with somewhat nationally represented sample. And what we asked was questions such as: “Are you on Facebook? Are you on this very dating site? How long have you been here?”, and so on and so forth.
So what we found is that for the people in the city we were studying, all the people who were on the dating site were also on Facebook, although the sample size there was pretty small. Across all our subjects, only 3% were on the dating site.
Nationwide we got similar numbers in the sense that 90% of the people who admitted being on the dating site were also on Facebook. And about 4% of all our subjects were on this particular dating site currently. If we included those who mentioned that they had been in the past on that dating site, the percentage goes up to 16%.
We didn’t ask for actual names because this was all anonymous, but we did ask if they used their real first and last names on Facebook. So take it as you may, there is always an element of self selection in this kind of surveys, but the results we got is that 90% of our subjects said ‘Yes’, they were using their real names on Facebook.
About 90% of Facebook members claimed to use their real first and last names on FB
And this data matches pretty well the study that Ralph Gross and I did a few years ago. This one was limited on Carnegie Mellon University students, and we pretty much got the very same percentage – 89% of users. In that case, we were able to verify the numbers because we could compare the answers to the survey with the actual profiles existing on Facebook CMU network. So it seems to be a relatively robust percentage.
Evaluation
Notice that PittPatt had to do a pretty large number of comparisons because there were more than 500 million potential pairs, and we were comparing each photo coming from Facebook to each photo coming from the dating site. We considered focus only on the best matching pair found for the dating profile, meaning that you have an image from the dating profile and you can have a list of one thousand, long list of potentially matching Facebook profiles. So we considered only the very top, in the sense that image for the Facebook profile would have the highest matching score found by PittPatt.
The matching scores that PittPatt produces are between -1.5, which is totally not a match, like one black image and one white image; to 20, meaning pretty much the same JPEG file.
We crowdsourced the validation of PittPatt’s scores to Amazon MTurk1, because we wanted some external validation. So we basically created a script where we had MTurkers who could not have known where this data came from, there were no names of course attached to the images. They had to rank it on the scale 1 through 5, meaning that the matches found by PittPatt were: definitely a match (1), likely a match (2), unsure (3), likely not a match (4), definitely not a match (5).
As you know, there is quite a bit of research of using MTurk properly because some people on the MTurk are very good diligent workers, and some people are just unbelievable cheaters. So there are a number of strategies that we have developed over time to cut out the cheaters. In addition to the traditional strategies that are used, such as trick questions before the survey even starts, what we also did was we inserted test pairs – definitely good matches and definitely bad matches. And if an MTurker got any of them wrong, we would kick that out, in the sense we wouldn’t consider their results in our evaluation. We also had at least five graders grade each pair.
Results
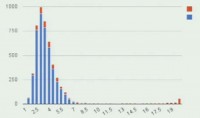
Here we have a histogram depicting the distribution of scores by PittPatt in blue, and the percentage of those potential matches which the MTurkers believed were a sure match – in red. So what you see are values basically from zero to twenty. And you can see that most of the action is happening in values between 1 and 7. The highest scores are the ones which are sure matches, and in fact red covers all of that. As you start going down in the scores, the ratio of blue increases because that means that more likely those are not real matches found by the recognizer.
At the end of the day, this is what we found: sure matches 6.3%, sure matches + highly likely matches 10.5%. What does it mean – sure or highly likely? Sure is if 2/3 of our MTurk graders gave a ranking of ‘This is a sure match’. Highly likely is if the majority of our graders gave a rating of ‘Sure match’. So about one out of ten users of the dating site were potentially identifiable.
About 1 out of 10 dating site’s pseudonymous members is identifiable
Comments
Now, consider that we only used one single Facebook photo, as I mentioned earlier, only using the primary profile image coming from a search engine search. And we only considered merely the first results found by the recognizer, rather than for instance the top ten. This is very important because these days recognizers can often be faked by some false positives. Sometimes the difference between two images is so little that maybe your best, your real match is the second or the third, or the fourth. Here we focused on the first only. But next, in experiment two we considered larger and more different models of attack. And of course recognizers’ accuracy will keep increasing.
3 photos per participant profiles
261,262 images
114,745 unique faces
Process and ground truth
And the process was like this: the subject would sit at the desk, and as the photo was taken, the subject would start filling out the survey about Facebook usage. Meanwhile, the image was sent up to a cloud service, a cloud computing cluster where the shots taken were matched against Facebook images.
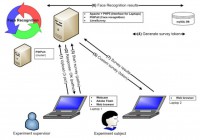
By the time the subject would have reached the last page of the survey, that page had been dynamically populated with the top best images found by the recognizer. And the participant had to click on “Yes, I recognize myself on this photo”, or “No, I do not recognize myself”.
So this is the schematics (see image): we had two laptops – one laptop was for the subject who was filling out the survey, the other – for the experimenters because from here we were sending the image to the cloud and getting back the results.
Results
93 subjects participated in this experiment. The survey that they filled out told us that they were all students, and they were all Facebook members, and for 31% of them we did find indeed a match.
One out of three were identified in this way, including one subject who told us right before starting: “You will never find me”. The reason was that he didn’t have a photo of himself on Facebook, or so he believed. And this is an interesting story. We found him through an image made publicly available by one of his friends. And the biggest story here is that it’s not just how much you are revealing about yourself, but it is also how much your friends or other people are revealing about you.
The average computation time was less than three seconds. It took much of this time for the recognizer to find the match than for the student to fill out the survey and arrive to the last page with the matches.
Pushing the envelope
And then we wanted to push the envelope, because okay, face re-identification is interesting, but the story we want to tell is bigger than this. What we found here so far is that we can take an anonymous face online on a dating site or offline in the street, and we can find the Facebook profile which arguably for 90% of people should give us their name.
However, two years ago when we were at Black Hat, we presented a study where we showed that we could start from a Facebook profile and end up predicting people’s Social Security Number.
So as a visual aid, what we have done so far is we started from an anonymous photo, we used PittPatt and found a Facebook profile. But two years ago, we started with a Facebook profile, we took the Death Master File, which is publicly available information, and we ended up with someone’s Social Security Number. So you are seeing where I am going with this.
As a quick parenthesis, what we did two years ago was we showed that Social Security Number had been misinterpreted by public for many years, meaning that people believed that there was much more randomness than there really was.
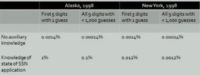
If you tried to predict someone’s SSN with the knowledge that existed before we published the paper, it would be really hard – in the sense that the probability of getting the first five digits right with one guess for someone born in Alaska in 1998 would be 0.0014% if you don’t know where they were born; and 1% if you do know. The probability of getting the full nine digits with less than one thousand guesses would be also extremely low – like 0.00014% (see image).
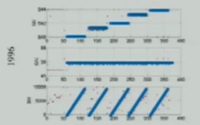
What we did was we took the Death Master File data, the data from the SSN of people who are dead, and we organized it chronologically. The X axis here for Oregon and Pennsylvania for people born in 1996 – represents date of birth, and the Y axis represents the area number (the first digit), the group number (the mid two digits of your SSN), and the serial number (the last four digits). So if you organize it graphically, you start seeing very obvious patterns such as group number remains constant over a period of time for people born in the same state over a certain period of dates; area number cycles in stepwise sequence; serial number increases linearly (see graph above).
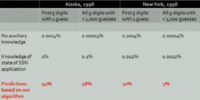
And with the help of all this we found out that accuracy of our predictions was much higher not just for the first five digits but also the last four than what was believed before (see image)– so much higher that brute-force attacks on SSN were indeed possible.
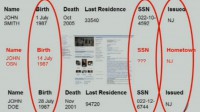
So this is what we wanted to do in our study. We took data from the Death Master File – say, John Smith born in July 1987 in New Jersey, and John Doe also born in July 1987 in New Jersey. And we compared it with John OSN (Online Social Network), who is not dead and is not in the Death Master File but happens to be also born in New Jersey, and also born in July. And by the process of interpolation we were able to end up predicting the Social Security Number of John OSN using the data form the other Johns who were instead dead (see image to the left).
Going back to our current study, we take someone’s face, anonymous, then we find their Facebook profile, and we end up predicting the SSN. So the story is predicting SSN from faces. But once again, this is just an example. I want to stress the reason we are doing this is really to think in terms of this blending of online and offline data, which I believe is inevitable five to ten years out.
What we did was we tried to predict from faces not only the SSN but also the interests of the subjects, we also wanted to add something easier to predict and less sensitive. So we asked participants in experiment two to participate in a new study. We used their Facebook profiles to predict their interests and other personal information.
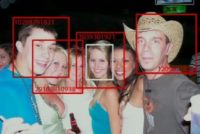
And this is really a picture which tells a story, sometimes they say: “An image is worth a thousand words”. So this is a little script that we wrote to in a way visualize Facebook tags (see image). This is an image where the tag linking to a certain Facebook ID (the numbers you see) is corresponding to a certain face. This is pretty much what happens when you are tagging people on Facebook: you are effectively creating these boxes with numbers on them.
We are visualizing it because the story we want to tell is that these red boxes are what faces were put, actually what you put through tagging. The white box is what our identifier found, for instance it found a match for this face. And because we have the tag information, we can find her profile, and from there we can find the personal information.
Participants of experiment
So we called back the participants of experiment two. Not all of them wanted to participate because some were understandably slightly freaked out by the study, although we put all possible safeguards in place. All studies were IRB1 approved, and no face, no SSN was harmed in the process of doing these experiments.
It was important for us that we would be able to get aggregate statistics of our prediction accuracy. We failed being able to know whether a certain given subject’s SSN was accurate or not, because we didn’t want to be in the position of inferring the actual SSN of a subject. So we had to run experiments in the way that we only would get aggregate data, which we did, and we also explained this to the subjects, but some of them didn’t feel sufficiently comfortable, so they started and they stopped after reading what it was really about.
For those who ended it, which was close to 50% of those who started, we got 75% of all their interests right. If we used just two attempts for the first five digits, we got 16.67% of the subjects right. If we used four attempts, we got 27.78% right. Now, this number may seem either large or low to you depending on where you come from, but I can tell you they are big, in the sense that if you use the random chance to predict the first five digits of someone’s SSN with a single attempt, the likelihood of getting it right, as I showed in previous slides, is 0.00014%.
Experiment three was obviously asynchronous, in a sense that first we did experiment two, in which in the campus building we took the photos and we found the profiles. Then we said, well, let’s push the envelope, let’s get more information from those profiles and let’s predict the SSN, let’s check with the subjects whether it is okay. But of course it’s more fun to do it in real time.
So we started working on a real-time application, a smartphone application which in real time tries to do what experiment two and three did, predicting personal, even sensitive information from someone’s face in real time on a mobile device.
Data accretion
So this is the story, and forgive me if maybe by now this story should be completely obvious, but because it is so important to me that not only the numerical results come out of this but really where we are going, I thought about using a graph.
The term ‘data accretion’ is a beautiful term that I found being first used by Paul Ohm. Many of you know Paul is a law scholar, an expert on anonymity at the University of Colorado Law School. He talks about data accretion to refer to this domino effect, when you start from piece of data A and then you infer data B, from B you can infer C, from C – D, from D – E, and eventually you discover something very sensitive such as SSN even though you started with something very innocent such as an anonymous face. Each step is obvious, but when you consider them as a whole – it is surprising.
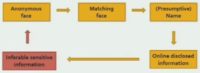
So we started from an anonymous face, we found the matching face; from here we get the presumptive name of the person. From the presumptive name, we use scripts to find additional information about that person, for instance from USA People Search, or ZabaSearch, or Spokeo. From this information we then used something to predict very sensitive information such as Social Security Number, which you can then reconnect to the original anonymous face.
The matching face, as I mentioned earlier, can come from LinkedIn or from Facebook profiles. The presumptive name is not always is so trivial, not always so easy. If you find a matching image from a LinkedIn profile, you are pretty sure that you have also found the name of the person. If you find a matching photo from Facebook, the story is a little more complicated than what I have told you so far. I have been hiding some important details, such as the following: the fact that if you find the face on a Facebook profile – you do not always know whether it is the face of the owner of the profile or of a friend.
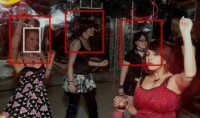
Imagine this photo (see image). Let’s say that I find a match for the girl on the left, and this is the only photo I can find for a certain given profile. It is a primary profile photo of Mary Johnson. Is that Mary Johnson? I don’t know because I cannot access additional photos about her. So how do we deal with the identification of the name starting from a matching photo? Well, it is not obvious, so we tried to write some algorithms for that. Then we tested the performance of the algorithms against human beings.
So we took 433 templates randomly chosen from our set of Facebook profiles, and then we asked humans to find who they thought these persons were in our script. And the script that we developed is based on the combinations of different metrics such as the following. Does the template, so the face, have a tag? If it has a tag, usually the tag is very accurate. Is it in a primary profile photo? Is it in a cluster? When you do cluster analysis and combine many faces together, you see the larger cluster inside a Facebook profile, under the assumption the larger cluster is usually the one of the owner of the profile.
Human coders were able to be sure about the correct Facebook profile in 46% of the times of the sample. The computer script we wrote was able to approximate human behavior 63% of the times.
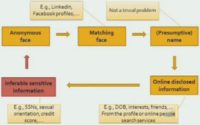
So going back, you go through this process and you try to find the presumptive name. Once you have the name, you try to find additional information about the name. In example I am about to show you, we used for instance USA People Search which is an online people search service that can be used for free if you use it only moderately, or for pay if you want to use it often. And then, from here you do the predictions such as SSN, sexual orientation, credit score and so forth. We also call it the ‘transitive property’ of personal information, when you go from A to B, from B to C, and so forth.
Real-time demo
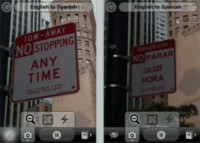
The real-time demo – what it eventually does is it also overlays the information obtained online of the image of the individual obtained offline. And this is the story of augmented reality. Augmented reality is really cool because you start seeing these amazing applications developed for smartphones. There is an application which uses your GPS data and when you are pointing in a certain direction it overlays information taken online to what you are seeing offline in the real world. Another really cool application is called ‘Word Lens’, I recommend everyone to check it because what it does is when you point it at text in Spanish, it translates it on your screen to English, and vice versa (see image). This is really cool but of course we wanted to see whether you could use it for our purposes.
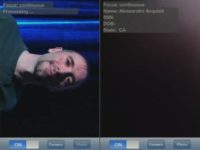
So we take a shot of someone’s face, and then we overlay on that face the name, the SSN, and their interests. The nickname of the project is the Wingman or the Wingwoman. Let’s test it on me. So what happens is that the image is sent to a server with a database of images. These images have the names, and the server looks for the name online and queries the service called USA People Search where, as you see, it correctly found that my first residence in the United States and where I got my SSN was California. Thankfully, it didn’t find when, so you can see the date of birth is empty and therefore there is no prediction for the SSN (see image).
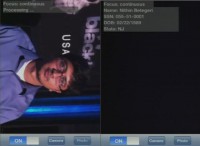
Let’s do another try with someone else’s face. As you see, it identified the person as Nithin Betegery (see image). In the case of Nithin, this is a real SSN so you can go and apply for credit cards on behalf of his name. Actually it’s not, because in the room full of you, we didn’t want to do it the real way. So we cheated with the last step, the Social Security Number is hardcoded, and if any of you is a Social Security Number nerd, you may have recognized that was the very first SSN ever issued by the Social Security Administration. Actually, Nithin has developed this application.
Now to where we were, I’ll tell you what was happening in the real time and what was not happening in the real time. So we were taking the photo in real time and sending it up to server in real time. Matching the face was not happening in real time, in the sense that we had hardcoded the database of images. We downloaded them from the Internet and we saved them. In the future, this can be done in real time easily, I believe, and I’ll tell you why a bit later. Getting presumptive name was done with a script in real time. Online disclosed information was found in real time, in the sense that the script was really querying USA People Search and finding my California residence. And SSN prediction was kind of done in real time, in the sense that it was, but of course we didn’t show the real SSN prediction, we hardcoded the fake SSN for Nithin; but the engine works in real time, the SSN prediction engine works in real time.
Limitations
So let’s overview the limitations now. Currently there are many limitations. What I have just showed is merely a proof of concept. Facial recognition is not yet there to a point where you can really recognize everyone everywhere all the time, but I am afraid that it is going to get there. There are technical limitations and legal limitations to creating large databases of images: computationally – because you need to download massive amount of data; legally – because, well, we use publicly available information; if you want more you start getting into Terms of Service problems.
Cooperative subjects – in our experiments people were showing their frontal face. Recognizers notoriously get worse when you use non-frontal faces for instance. And of course if you try to identify someone in the street, we found it’s a little difficult to take nice frontal face photo and not be identified yourself.
Geographical restrictions – experiments one and two were about specific communities, large (hundreds of thousands of people) but not all the United States. The larger you go the more computation time is needed to do the face comparisons, and of course the more false positives you get from that process.
Extrapolations
Even though, clearly, face re-identification everywhere, anytime, anybody is not yet reality, this is where we are going. And why am I saying and thinking this? Well, yes, there are existing legal and technical constraints, however many sources of public data with identified facial images are already out there. I would argue that for most of us in this room – there are. Tagging self and others is absolutely socially acceptable and has become very, very common. And in fact there are companies such as Face.com which collaborate with Facebook, and they are discussing, almost boasting on their website how they have already tagged and identified billions of images. So if someone, a third party doesn’t do it based on publicly available information, someone from inside one of the big companies will do it.
Availability of images
I do believe that visual facial searches will become more common. What I mean by this is currently we have text based searches – search engines index text, but they can well index images. And in fact, Google recently started talking about pattern-based image search, although not yet facial images but we are really going to get there. Think about 1995. In 1995 the idea that someone could put a name Alessandro Acquisti in something called a search engine and find all the documents on the Web with the name was unthinkable. Maybe five years from now visual searches and facial searches of the type I am describing will be as common, even though they sound as unthinkable now as text-based searches were ten years ago.
And really, if you see the trend I mentioned earlier with Apple, Facebook, and Google going on a shopping frenzy on startups doing face recognition, you obviously see where the commercial interest is, it is not going to stop.
Cooperative subjects
So the other issue is cooperative subjects. It’s true, our subjects were cooperative. In experiment two we had nice frontal photos, in experiment one we had dating site profile photos where people usually use frontal photos, and sometimes they use the artistic shots, and those ones are faces too. But then again, you can take frontal photos also without being noticed. You can use glasses, there is allegedly the Brazilian police already deploying this for the World Cup in 2014. How long will it take before it can be done on contact lenses? Of course it is impossible to develop now, but five to ten years out contact lenses that tell you in the street what is the last blog written by the person you are just walking by. Is it so unthinkable? I don’t think so.
And then, face recognizers keep getting better, also detecting non-frontal photos. As we were doing these experiments, a new version of PittPatt came out. We started with PittPatt 4.2, we now got PittPatt 5.2; we noticed just in the space of ten months of developing of PittPatt a dramatic increase in the ability of the recognizer to detect and recognize side photos.
Geographical restrictions
Geographical restrictions again – the point is that we were using data on hundreds of thousands, but nevertheless a specific community, while if you want to do it on a nationwide scale, hundreds of millions of people, the computation time increases and the number of false positives increases. This is absolutely true, although cloud computing keeps getting faster and larger. More RAM means larger database you are able to analyze, as well as if the accuracy keeps increasing, the number of false positives keeps decreasing.
By the way, something else that we discovered as we were doing these experiments was that in reality computers aren’t so bad recognizing images – we humans are also very bad, in a sense that when we put a human in a task where the only information they have is two photos of the face of a person, I can tell you it’s really hard for some people to recognize.
We humans are so good at recognizing each other because we use all additional contextual clues such as the body shape of the person, how they walk, maybe how they are dressed. Holistically, we capture this information instantly and we use it to say that is Richard, for instance. But when we only have a shot of a face, our ability of recognizing faces decreases, it is not just computers. And you know what – online social networks are going indeed to provide these additional contextual clues.
Implications
So this is why at the start of my talk I was mentioning this idea of Web 2.0 profiles as de facto unregulated Real IDs. And interestingly, the Federal Trade Commission1 some time ago approved the Social Intelligence Corporation, a company which wants to do social media background checks. This suggests, really, this idea that we are starting accepting online social network profiles as the real deal, as the real veritable information about the subject.
Now, this creates cool opportunities for e-commerce. Imagine the first picture I showed from ‘Minority Report’ movie, where, say, the ‘Gap’ company in the street can see you enter the store, connect your information from online because on Facebook you are member of the ‘Gap’ fan club and so forth. And you don’t have to wait until 2054 for this; it will be five, ten years from now or less.
But there are really also ominous implications for privacy. Once again, I am a privacy researcher. I am not in this to make a startup and allow companies to track people. I am here to raise awareness about what is going to happen, what I feel is going to happen.
And I feel that this is very concerning. And the reason is that if you consider the literature on privacy, in fact also the literature on anonymity – it is well known by most of you in this room – we are told to expect that anonymity loves crowds. We are anonymous in a crowd; our privacy is protected in a crowd. But here we have the technology which is truly challenging our perception, as well as our expectations of privacy, in a physical crowd in the street, because a stranger could know your last tweet just by looking at you, and online on a dating site, on Prosper.com and so forth.
We don’t anticipate this because we are not involved to think that strangers can recognize us so easily. Not only that, but I know from my other similar researches which are about behavior economics, that we cannot really anticipate the further additional inferences which become possible once you are re-identified.
The problem is that there is no obviously clear solution to meet this problem that doesn’t come with huge unintended consequences or simply which doesn’t work. The ones which do not work are for instance opt-in or user concern; I find them ineffective because most of the data is already publicly available: Facebook for instance – I was mentioning earlier the case of primary profile photos being by default visible to all. Regulation? But what type of regulation? Do we want to stop researching face recognition? Obviously not, there is so much good that can come out of that.
Finally, one of the two final questions is: what will privacy mean in this future of augmented reality where online and offline data blend? But not only that, if you allow me to extrapolate a little widely, to be a little bold, what else will it mean for our interactions as human beings? What I am talking about is the fact that we have evolved for millions of years to trust our instincts when we meet someone face to face, immediately. Our brain, our biology, our senses tell us something about that person: whether the person is trustable or not, whether we like that person or not, whether that person is young or old, cute or not cute and so forth.
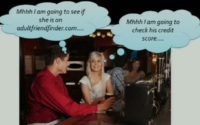
Will we keep on trusting these instincts that evolved over millions of years? Or will we start trusting more the technology, our contact lenses, our glasses that, as we look at the other person, tell us something about that person?
I will go now with a stereotypical joke (see image). This is the Wingman application of face recognition. A guy and a girl meet at the bar, and of course they are using their iPhones to check each other out. And the guy is thinking: “Mhhh, I am going to see if she is on adultfriendfinder.com”. And the girl is thinking: “Mhhh, I am going to check his credit score”.
Our surveys
So, interestingly, in the survey we ran we asked our subjects both of experiment one and experiment three: “What do you think about this?” Before we had identified them we asked them: “What do you feel about the scenarios where people in the street, complete strangers could know from your face your credit score?” Almost everybody was freaked out. At the same time, almost everyone is revealing online the information which is precisely the information we used for this prediction. So we once again have this paradox. I am not the first one to point it out, the paradox between attitude and behavior. But in a way, I fear that this blending of online and offline data that face recognition, cloud computing, online social networks and statistical re-identification are making possible, is pushing the paradox to its most extreme point, which is the unpredictability of what a stranger may know about you.
Key themes
And that’s why I want to conclude with the themes I started from, because I really hope that the big story here is not the numbers but what is going to happen five – ten years out.
- Your face is the conduit between online and offline world
- The emergence of ‘personally predictable’ information
- The rise of visual, facial searches: search engines allowing people to search for faces like today you search for text
- Democratization of surveillance, which is not necessarily a good thing
- Social networks profiles as de facto Real IDs
- The future of privacy in this augmented reality world
1
A tale of two futures
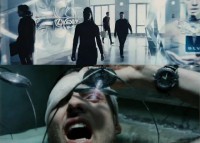
So let me conclude suggesting that of these two futures (see image) one is definitely creepy, perhaps both are creepy, in a sense that personally I don’t want to live in a world, if I had the choice, where anyone in a bar can know my name. If I go to the bar I like them knowing my name if they are my friends, not everyone. But we don’t know which kind of future we are walking into, so we had better be prepared, and this is why we are doing this kind of research.
Thank you!