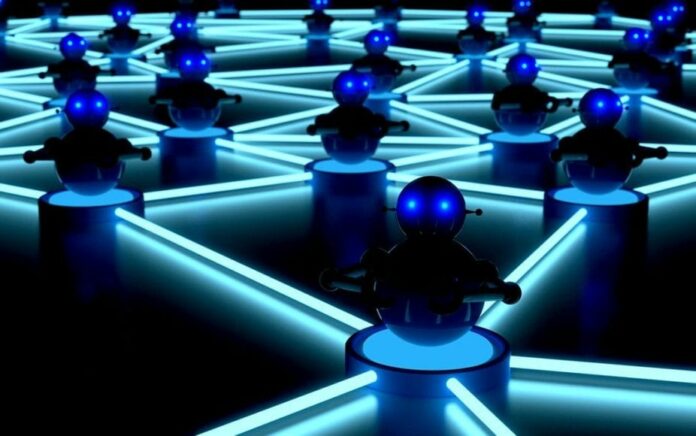
CrowdStrike’s researcher Tillmann Werner provides an extensive overview of peer-to-peer botnets, covering the essentials and architecture details thereof.
Welcome to my presentation! I’m Tillmann Werner; I work for a company called CrowdStrike which is an American startup that deals with targeted attacks. But today I’m going to talk about something else; I’m going to talk about one of my favorite topics, one of my hobbies, which is peer-to-peer (P2P) botnets. And peer-to-peer botnets are interesting because they are designed to be resilient against attacks; and I’m usually trying to attack botnets and have fun with them.
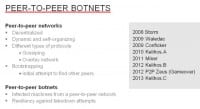
Let’s start with a quick introduction to peer-to-peer botnets. I guess most people in the room here are familiar with peer-to-peer networks in general; those are networks like BitTorrent, eDonkey and other file sharing networks. Usually the purpose is to build a decentralized infrastructure that’s self-reorganizing, so if parts of the infrastructure go offline it recovers itself. People usually build peer-to-peer networks because they want to get rid of any central components so that the infrastructure cannot be taken down so easily.
When you analyze a peer-to-peer network of some sort, you want to understand the protocol first. That’s not too much of a problem for all the popular file sharing networks because they are well documented, but if you look at peer-to-peer botnets they usually use their proprietary protocols that you have to reverse-engineer and understand first: you have to look at the samples, do the reverse-engineering, and so on. But if you do that for several peer-to-peer networks you will at some point see that there are different approaches.
One is based on gossiping. If you think about that, you have all these different nodes that are interconnected somehow and you want to propagate some information in this peer-to-peer network. You can do that by what we call ‘gossiping’, where each peer kind of gossips information to its neighbors, basically forwards information to all its neighbors which in their turn do the same. But if you think about that, that’s probably not very effective, because probably several peers will receive information several times, so you will fill up the network with more information than you actually want to or have to.
So, more advanced P2P networks use what people call an overlay network, where you have addressing on top of the general addressing methods like IP, so every peer has an ID or some sort of address, and then there is a routing method so you can address specific peers. If you want to send information to a specific peer, then if you know its address you can route that through the peer-to-peer network. An example for that is eDonkey, where you have a distributed hash table on top of the IP network; every peer has a hash which is at the same time its ID, its address; and then you can look up data in the hash table and so on.
One important thing when we talk about peer-to-peer networks is bootstrapping. Bootstrapping is a process of establishing connectivity with the peer-to-peer network when a new peer comes online. That’s a very important aspect, because when you think about that, you want to get rid of any central entities in your P2P network, right? So it might not be a good idea to have a seed server that all peers contact to request an initial peerlist. That will be a central component you don’t want to have. So, what people are doing is they deliver a seed list of other peers together with a node itself, for example with an executable that’s executed on the node system.
But what happens if these peers go offline for some reason? Then you do the fallback method, and that’s where it’s getting interesting. If you look at the box at the right-hand side (see image above), the third entry is Conficker which is a very famous, or infamous, piece of malware that was active in 2009 and in the following years, and still is very active. Conficker used random scanning: it scanned the Internet for other peers randomly, and of course there’s no way to block that. There’s no information that the bot relies on when it’s first started; it just starts scanning the Internet until it finds other peers, and then gets other peers from that one, recursively, to establish connectivity with a network.
Speaking of that box on the image, that’s my own private history of P2P botnets I analyzed. So, I started in 2008 with the Storm worm which used the eDonkey network to get a list of other people. There are early peer-to-peer botnets that are known – I think Nugache was active in 2007, and maybe there were some others but I think Nugache from 2007 is the earliest I know personally.
Then there was Waledac which people believe is a successor of the Storm worm, because Storm called a lot of attention by researchers and lots of security people tried to investigate Storm and tried to understand the protocols; some even designed attacks – how you can attack a peer-to-peer network to knock it offline or take the nodes offline. So, apparently the people behind it decided to abandon it at some point and create a new botnet that was called Waledac which was not relying on any existing P2P infrastructures, no eDonkey anymore. They implemented their own proprietary protocol which – maybe I should not be saying this – was very similar to eDonkey, but the overall concept behind the botnet had similar structures and design characteristics, that’s why people said it’s probably a successor of Storm.
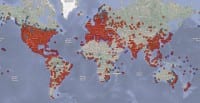
I already mentioned Conficker. Conficker was interesting because it started out as a bot that was entirely centralized with its command and control infrastructure. Many of you probably have heard about the DGA, the domain generation algorithm that it included. So it generated pseudorandom domain names all the time and then tried to resolve these and contact that host and ask for, basically, updates. Later on these people switched to subversion C, they switched to a peer-to-peer protocol as a fallback command and control channel because there was some effort to block access to the generated domains, so they needed something else otherwise they would lose their 8 million nodes botnet.
So, there was Conficker, and then in late 2010, I believe, the Kelihos era started. That’s a bot that’s also known as Hlux, which is the other well-known name. That, again, is believed to be a successor of Waledac, and that is because Waledac was taken down by some people and myself with a P2P poisoning attack, and I will talk about that a little bit more in a minute. So, that botnet was taken away from them, and they created a new one that was called Kelihos.A. And that’s actually interesting because, if you look at the list, Kelihos.A was attacked as well with success, so they created Kelihos.B, a successor, and tried to fix some stuff; that was taken down as well and, again, they created Kelihos.C, the third version. We attacked that as well, it wasn’t too successful because we didn’t manage to own all the peers.
And just recently they changed something in the protocol and added private-public key encryption to it, which doesn’t make sense at all because, you know, you might want to encrypt your traffic but you can’t do it with symmetric encryption. It doesn’t make sense to do private-public key stuff because the peers have to generate their own keys and exchange keys, and so on. I mean, anybody can do that; you can still infiltrate the botnet by just doing the same, so it doesn’t make sense.
And then, in 2011 there was the Miner botnet, and I will show you some protocol examples for that. A really stupid piece of malware that was written in .NET if I’m not mistaken, and the protocol was HTTP-based, so it was a plaintext protocol. They made several mistakes, so it was trivial to take down.
The remaining two, ZeroAccess and P2P Zeus, are somewhat interesting because they’re still around and they’re really successful. They’re some of the biggest and most prevalent botnets that are around these days, and they’re mostly used for dropping other malware on the infected systems; especially ZeroAccess – it’s basically a platform that is used to deploy other malware, like click bots, etc. ZeroAccess is actually split into, I think, seven or eight separate botnets. I don’t know why, maybe they have some affiliate program or something. They also distinguish between 64- and 32-bit systems, probably because they want to maintain two separate infrastructures.
Okay, going back to my slide here, obviously people build peer-to-peer botnets because they have the same goals as some other people who build peer-to-peer networks: they want to create a resilient infrastructure that is resilient against takeover attempts or takedown attempts. So, that’s the goal and that’s why they are getting somewhat popular.
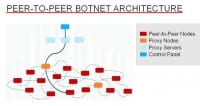
Interestingly, for all botnets that you’ve seen on the previous list the architecture is not purely peer-to-peer. It’s hybrid architecture. That’s what you see here (see right-hand image). The thing at the bottom is the actual peer-to-peer network, and the dashed lines represent a peer being in the peerlist of another peer. But when they want to receive commands for sending out spam or something like that, they still reach out to central components. And the boxes you see in the middle are proxy servers, they usually have another layer in between so that when some of the proxy servers get taken down they can easily replace them without losing their command and control infrastructure.
And then, there’s the command and control server on top – that is the actual backend. There might actually be multiple layers between the peer-to-peer network and the C2, but unless you get access to one of the proxy servers you don’t see what’s behind it. We are fairly certain that in most cases these are proxy servers because, you know, for example when they speak HTTP and respond with Nginx, then you can be certain it’s a proxy, most likely at least.
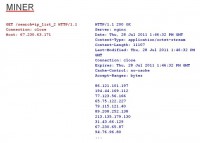
Okay, let’s take a look at some protocol examples so that you get an idea of what these people create and come up with. This (see left-hand image) is the already mentioned Miner bot, and as I’ve said, that was a really trivial and also stupid protocol. It was HTTP-based and all the bots implemented their own tiny HTTP server which was a very rudimentary one that was backed up by the file system. So, if you would issue a GET request with the ‘search’ parameter and the ‘ip_list_2’ value, that filename would be looked up in the respective directory and then delivered to the requesting host. If there were other files on the file system you could request them as well with this method, and that was probably not intended by them.
So, you can see the response here. In that case, I think the Nginx server header is fake, they just copied that from somewhere and sent it with the responses. And you can see at the bottom is the actual payload, a list of other peers, a list of IP addresses. Miner always responds with the entire peerlist that it has, all peers that it knows about. And that’s stupid because it can be huge. And also that makes it easy for us to enumerate the bots and understand how many infected machines there are if you want to attack it, for example. You can see it’s 11107 in size, and this is by far not the largest response we’ve seen.
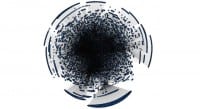
You can try to recreate this peer-to-peer graph (see right-hand image), because it’s basically a graph – it’s nodes who know about other nodes and talk to other nodes, and so on. You can try to recreate that graph by crawling peers, and we will talk more about crawling, that’s the topic of the presentation, right? If you request a peerlist from one peer you can recreate these links on the graph and then take the IP address from the response you got back and plot pretty pictures like this one here. I think that’s about 37000 nodes, which is only a subset of the Miner botnet at the time, but it takes ages to render this picture here, so we only did that for a subset of the nodes we found.
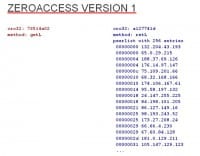
You can see that other P2P protocols are somewhat similar. This is ZeroAccess version 1 (see left-hand image); there are two versions out there, this is the earlier version. Again, it’s proprietary protocol that they implemented. They define, I think, six different message types, and one is a ‘getL’, which means ‘get peerlist from another peer’, and the ‘retL’ is the ‘return peerlist’ message. This is what you get when you reverse-engineer the message form and decode it. It’s not plaintext; I think ZeroAccess version 1 had a 4-byte key that it hashed with MD5 and then used that MD5 hash as an RC4 key to decrypt its messages, but it’s always the same key, so it was basically symmetric encryption with a static key. And version 2 just used XOR with another key.
So, if you undo the encryption you end up with something like this and you can see here, in the case of ZeroAccess version 1 a peerlist has 256 entries, so it always returns up to 256 entries. But since the botnet is large enough, every peer always has more than 256 entries at any time. So, whenever you ask a peer for its peerlist you will most likely get these 256 entries.
As you can see, there’s some order there. The first number is a timestamp, or time delta, so to speak, because the botnet favors peers that have recently been active. And that makes sense because you don’t want to keep the peers in your peerlist that might be offline already, or they reboot from time to time, getting your IP address, so the entry becomes invalid. So you might want to favor peers that have recently become online or that you have recently talked to; that’s why they sort this peerlist by the time delta and then return the 256 most recent ones.
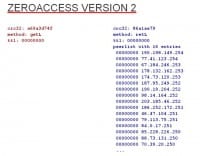
They changed this protocol a little bit in ZeroAccess version 2 (see right-hand image). You can see there, again, the two message types. I’ve already mentioned that the encryption is slightly different but for the most part the protocol is very similar. So there’s ‘getL’ and ‘retL’; again, you have the timestamps and you have the IP address. But they figured that they don’t need to send back 256 IP addresses, that’s way too much. It’s sufficient if you respond with only 16 IP addresses. That makes the messages smaller, hence less overall communication in the botnet. ZeroAccess version 2 is really huge. We’ve crawled some of the botnets, and they count around 3.7 million infected machines – I think it was Conficker. And if you have 3.7 million machines talking to each other, that’s a lot of traffic, so you might want to reduce the message size. That’s what they did.
If you take a look at the IP addresses you might notice that the last octet looks a little bit strange, it’s always very high. And that is because they do some deduplication. You don’t want two or multiple entries with the same IP address in your peerlist, obviously, because if you allow that it’s trivial for other people to poison your peerlist and inject one entry multiple times, overwriting all the legitimate ones, and then you are not connected to the P2P botnet anymore. So, that’s why they do deduplication, and in order to do that they sort the IP addresses, then go over the sorted list and if they have two consecutive entries that have the same IP address – they kick one out. But because IP addresses, at least on PCs, are sorted in little-endian, you have as a result these IP addresses with the high last octet in the response.
What’s interesting is that they do that but they don’t filter out invalid IP addresses. So, when you crawl the botnet you come across IP addresses like 255.255.255.255, which obviously is an invalid IP address, it shows up on this list because when you sort the list in decreasing order, it’s the topmost entry and it’s always included. And they have some other garbage in there; for some reason they don’t filter out these entries, which is interesting.
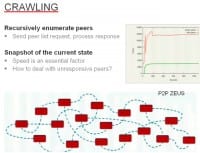
Let’s talk about crawling. Crawling is nothing else but recursively enumerating peers. You start with one peer, you request its peerlist, you take a look at the response and do the same for all the returned addresses and so on until you want to go offline or something like that. You really want to think about a crawling strategy, and one important thing is crawling speed. Ideally, we would be able to take a snapshot of the current peer-to-peer graph and then enumerate the peers in that snapshot, but that’s not possible. First off, because you have to do that actively, you have to send out requests and process the responses, and that takes time. And while you are doing that, the structure of the graph might be changing: peers might go offline, new peers might come online. So you will never be able to get that snapshot, but to come closest to that you want to be as quick as possible.
And when you do that you have to think about things like unresponsive peers: what if somebody sends you an IP address back that’s offline? How do you deal with that? Do you want to keep it in the list and try again later? I mean, you don’t know why it’s unresponsive, you might lose packets, the network might be overwhelmed with your traffic because you try to be as fast as possible. You don’t know why it’s unresponsive, so you might want to keep it in the list and try again later, but you can see it’s getting a little bit more complex.
What you see in the top right corner (see image above) is the result of us crawling P2P Zeus, which is also known as Gameover by the way. The red line shows you the number of IP addresses that we learned, we call them known peers, but most of them are not actually reachable although the protocol is pretty robust and they don’t include any invalid IP addresses in it. But most of them are not actually reachable. So, if you count only the peers that you can talk to you end up with the green line, and you can see it’s way less. If you see these little dips in the red line, that’s because for P2P Zeus we chose the strategy where we cleaned up the list of known peers from time to time, so we said: “Okay, these are unresponsive for too long now, let’s kick them out to keep the list small.” Because otherwise you’ll have an endlessly growing list. What you can also see is that the green line converges very quickly, and that means you have probably reached the number you are able to crawl, and that gives you some size estimation.
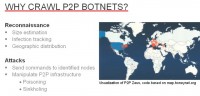
You might wonder why anybody wants to crawl P2P botnets at all. It’s interesting to play with that, it’s interesting to understand the protocol and re-implement it and so on, and then play with the botnet and maybe snoop on what they are doing. But we usually have other goals. Reconnaissance is usually the foremost thing, right? But why would you want to learn something about peer-to-peer botnets and the infected machines? I’ve already mentioned size estimation. If you talk to the press, they really like high numbers, so if you tell them that, you know, ZeroAccess is 10 million infected machines large, they will love that, but next time you have to tell them the botnet is 15 million infected machines large or something.
So, yes, size estimation is one thing, but you have to be aware that you can only crawl a subset of the infected machines. Most of them, obviously, are behind NAT, behind gateways. You can’t directly talk to them, you can’t reach from the Internet, but they’re still part of a P2P botnet, they are like leaf nodes in this graph. So, it’s not trivial. If you do what we did with P2P Zeus and you end up with this green line and you get the number of machines that you can talk to, you have to extrapolate from that number to get to a more realistic size estimation.
Infection tracking is something being done by the people who want to remediate or kill these botnets. They want to learn about infected machines and then can report the IP addresses to, let’s say, ISPs who then pass the information on to their customers, and hopefully they clean up the machines so that the botnet dies off, but I’ve never really seen that being successful.
Geographic distribution is something you can also get from that. If you have all the IP addresses you can do geolocation lookups and, if you want to, plot them on a map like what we did here (see left-hand image above). And I want to mention Mark Schlosser and some other guys who created the code we based this on. This is actually a live thing, so we send it a live feed of the crawling results and that displays these little red dots.
Okay, what we are usually after is we want to attack peer-to-peer botnets, so if you know all the nodes you might want to try and send them commands yourself, sometimes interesting commands like uninstall command: you send an uninstall command to all the bots you’ve identified, and they are the ones you can talk to, so it’s the backbone of the whole graph, so to speak – then you can kill the botnet entirely. Or if you can send requests for more information about the infected machines, you can for example get information about the operating system version or other stuff, that’s usually interesting as well.
You can also manipulate the peer-to-peer infrastructure. Think about it: if you can generate your own peerlists and then propagate these into peer-to-peer network, you can, basically, tamper with that infrastructure, and we will talk about that in a little bit. Ideally, you might be able to sink the whole thing by replacing all the legitimate entries in the peerlist with your own ones and by that have all peers talking to your machine, which means that nobody else has access over them anymore.
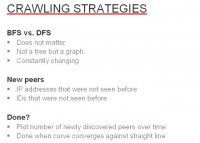
If you think about crawling strategies, you might ask yourself: “Do I want to implement a depth-first search (DFS) or breadth-first search (BFS)?” It doesn’t really matter, at least that’s what we think, because, first off, it’s not a tree, it’s a graph; I mean, you can distinguish the two strategies anyway, but it doesn’t really matter because it’s dynamic. It’s changing all the time anyway, so it doesn’t really matter which nodes you start with and which nodes you continue with. At some point, if you are quick enough, you will hopefully be able to learn the biggest part of the reachable machines.
If you track the infected machines you need to be able to distinguish: “Have I seen that IP address, or have I seen that peer before? Do I want to include it in my lists?” And if you rely on the IP addresses only, that’s a bit of a problem because, as I’ve already mentioned, there’s a lot of IP churn – you know, IP addresses that change after 24 hours; and if you happen to crawl a peer and then the IP address changes, you contact it again and you count it twice, so you want to avoid that otherwise you get screwed numbers.
Some P2P protocols are nice, they implement unique IDs, especially ones that implement overlay networks because you need them for routing. And if you have that, then you can have more accurate numbers. When you’re done with the crawling and this curve converges because you don’t learn about any new peers anymore, and if there are some changes – then it’s due to churn.
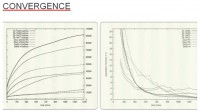
What you see here is analysis of the convergence for the P2P botnets we crawled (see right-hand image). On the left-hand side, you see a curve similar to the one on the previous slide, reflecting the actual number of the machines that we identified. The upper curve is ZeroAccess which, as I mentioned, is pretty large and gets way more hits. And the one at the bottom is for a botnet called Sality, which I haven’t looked into myself but one of my friends has and he has provided these numbers. Depending on the size of the botnet, the scale is different, but the shape is more or less the same: you can see that all of them kind of converge. When it’s a straight line, then you know you are pretty much done. You can also take a look at the population increase, that’s what’s displayed on the right-hand side, which basically correlates with the other graphs.
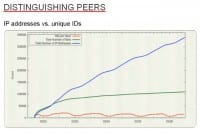
So, how do you distinguish peers? I already talked about that: you have unique IP addresses vs. unique IDs, in the case where you have IDs. In the case where you haven’t, you can still derive some conclusions from other cases where IDs are available. I’m cheating a little bit here because these graphs are not generated by crawling; this is the Kelihos.C botnet (see left-hand image), the last version that was attacked. These numbers are not generated by crawling the botnet, but in this case we did node injection, so we propagated a special peerlist entry in the P2P network, it came very prominent, and then all the other peers reached out to that machine. By this you even get the ones that are not directly reachable, because at some point the entries propagate through NAT and through gateways, and so on.
So, this gives you way more accurate numbers, and that allows us to compare the IP address count with the ID count. Green here is the total number of bots, total number of unique IDs; and blue is the total number of unique IP addresses, and you can see that this goes up even though we have seen almost all unique IDs, so the slope is much slower for the green line. The ratio between the two after, say, 24 hours or 48 hours is almost the same for all botnets we’ve taken a look at.
So you can see after 24 hours, that’s where the two lines cross. Even if you do not have unique IDs you can say: “I take a look at the IP addresses I collected for 24 hours, and that gives me probably pretty accurate numbers.”
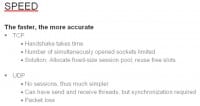
I already mentioned speed. Speed is important, you want to be as fast as possible, but being fast is not easy (see right-hand image). I mean, if the protocol is UDP-based it’s a little bit easier because you don’t have to worry about session establishment, timeouts, etc. Most of these botnets use UDP for a reason, it’s way simpler. Usually people have either two threats – one that sends out messages and one that consumes incoming messages – many bots work that way, actually most of the UDP ones we have seen. If you do that you have to worry about synchronization, so you have to have a peerlist that you lock when you want to send out stuff, or when you receive data you also probably want to lock the peerlist. So you have to synchronize the two.
When you are talking TCP, it’s a little bit more difficult. You have to establish TCP connections and you have to worry about timeouts because you don’t want to get DoS’ed, right? If you don’t worry about all these things and you crawl the network, they might create half-open connections and not respond to you at all or keep established connections open forever; and then you’re running out of file descriptors and your crawling doesn’t work anymore. So you probably want to have a limited set of file descriptors, or sessions that you are able to handle. What we do, what the code does is it allocates a fixed number of slots for sessions, and that’s the amount of simultaneous sessions the code can handle. And when it wants to contact a new peer, it takes a next free slot from that array. By that you make sure that your crawler doesn’t get DoS’ed.
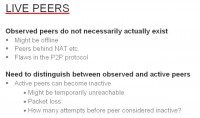
Another thing is if you talk to a peer, then you can definitely say that it’s live, that it exists, and the question is how long you want to keep it in your peerlist flagged as active (see left-hand image). As I said previously, you want to distinguish between IP addresses, or peers, that you have encountered, and the ones that you can actually talk to, that are live. If you talk to a peer that’s live, for how long do you want to consider it live? That’s another thing. I mean, do you want to consider it live for 24 hours or only three minutes; or do you want to periodically re-contact it and if it doesn’t respond anymore, then you say it’s not live anymore? So, these are parameters that are really important. Might not sound like that, but they are really important and you might want to tune them for the specific botnet that you’re crawling to get accurate numbers.
Especially when you’re talking UDP – you can send out lots of UDP packets per time, and if you fill up your own line with UDP packets, you will have packet loss sometime and then you get funny results: either get a bigger line, bigger bandwidth, or slow down a little bit. So you want to have a parameter that allows you to slow down the whole crawling process.
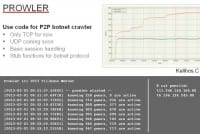
So, Prowler is the name of the tool that was recently released (see right-hand image). As I said, it just implements the crawling framework, so to speak, and you have to add the protocol implementation yourself. It provides you with some stub functions that get called, and that’s where you have to implement the protocol. So, if you want to check it out, please do. It’s only TCP for now. You can see what it looks like at the bottom of the slide; you can even see that it distinguishes between known peers and active peers. If you take a look at the last two lines, you can see that the number of active peers goes down from 719 to 717, and that is because after some time some peers don’t respond anymore, so they are not considered active anymore and get flagged as inactive.
In that case, we were crawling Kelihos.C and that was in February. The peerlist I started off with only contained two entries, you see that on the right-hand side. And Kelihos always shares 250 entries, and that is why, if you take a look at the first line – it immediately goes to 250 known peers. It contacts one peer, it learns 250 entries, so it knows 250 other ones immediately, and then it continues from there. But if you take a look at the two graphs, again, the green line is active peers that you can talk to, and the red line is peers that I have seen in peerlists. You can see that the green line gets constant very quickly, so it converges really fast. And that is because Kelihos also favors more recent peers, so they have this backbone of what they call ‘router nodes’, and there’s never more than in the range of 700. That’s why we’ll never be able to talk to more than around 700 peers at a time.
You can also see these sharp rises, or steps, on the red curve, and that is because if new peers come online they propagate in the peer-to-peer network, become active at some point, and then you get these steps – when a new peer comes online it immediately propagates to all peers that are online, and that’s what causes this effect.
I’m almost done here. This is the repository where you can check out the code (see URL above). As I’ve said, I will hopefully add a UDP version soon.
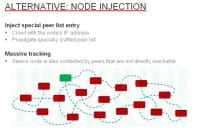
I would also like to talk about the alternative that I’ve already touched upon briefly, which is node injection (see left-hand image). By crawling, you will never be able to reach the peers that are behind gateways, network address translation, and so on. So you can actively participate in a peer-to-peer network and propagate your own IP addresses, and then at some point, depending on the popularity of your node, the other peers will reach out to you and say: “Take me down”, or “Send me commands”.
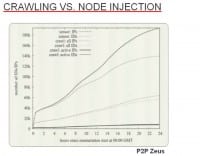
That’s actually a comparison here between tracking based on sensor injection and crawling (see right-hand image). Again, this is P2P Zeus, we have unique IDs and IP addresses – of course the number of IP addresses is much higher. The top two lines are what we achieved through sensor injection, and the other lines are what we achieved through crawling. The bottom lines are the active IP addresses, or the active peers, that we can talk to, so you see it’s much less than the peers that show up in the peerlist.
Okay, that’s basically it for my presentation. Thank you!