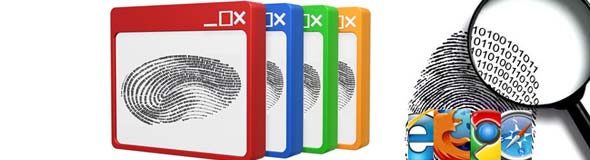
Technology Projects Director for EFF Peter Eckersley speaks at Defcon 18 on methods for identifying a person based on different sets of information, including data like one’s ZIP code, birthdate, age and gender, gradually shifting the focus onto some computer-based criteria such as cookies, IP address, supercookies and browser fingerprints.
Hello everyone, so today I am going to talk about browser fingerprinting, in particular about an experiment that we did at EFF1 to measure how fingerprintable web browsers work, called ‘Panopticlick’.
So before we get to browsers, let’s talk about identifying information. Now, when we ask what kind of information identifies a person, we have some standard sorts of answers, like if I know their name and address, I probably know who they are.
But there are some more surprising examples of identifying information. There’s a paper by Latanya Sweeney2 written in the 90s, stating that if you know someone’s ZIP code and their date of birth and their gender, then you have about an 80% probability of being able to identify them uniquely. Well, that’s a bit surprising, so let’s see how it happens.
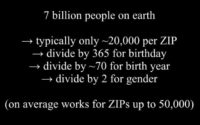
And it turns out that if the ZIP code you started with had fewer than about 50,000 people in it, you now probably have a unique person at the end of this process.
So there is a mathematical measure you can use to say how identifying a set of facts about a person is, or how much information is required to identify someone. And if you need more bits to identify them, then each bit doubles the number of possibilities. And if you are learning more facts about them, then each bit you learn halves the number of possibilities, so you can think of these as trading off against one another.
So for instance on the face of the planet Earth with 7 billion of us people, you need about 33 bits to learn the identity of one of us. And if you learn someone’s birthdate – what day of the year they were born on – you learn about 8.51 bits.
To identify a human, we need: log2 7 billion = 33 bits
Learning someone’s birthdate: log2 365.25 = 8.51 bits
So if we talk about a random variable you might measure, like someone’s birthdate, you can talk about the amount of information you learn when you learn a particular value of that random variable. So if we are talking about birthdate, you learn that my birthdate is the 1st of March, then you’ve learned 8.51 bits about my identity. If, however, you learn that my birthdate is the 29th of February on a leap year, then you get a bit more information because the likelihood of that being true is only a quarter of what it would be for any other birthdate, so you get more information – 10.51 bits typically.
So we call that first measurement the surprisal, or self-information of the fact you’ve learned. So surprisal that I was born on the 29th of February is 10.51 bits. And then we can talk about the entropy of this type of measurement, which is the expectation value of the surprisal. So if you have a probability distribution, you measure the expectation across all of that.
Birthdate = 1st of March: 8.51 bits
Birthdate = 29th of February: 10.51 bits
A point to know is that you can’t add surprisals together. If you learn someone’s birthdate and then you learn what city they were born in, those two things are probably independent variables – not entirely but close to. So you could add the number of bits together. But if you already know someone’s birthdate, and then someone tells you their star sign, you are actually not going to learn any more information. So if you want to know how two of these measurements add together, you need to do some fancy stuff with conditional probabilities.
So what use is all of this theory? Well, we can apply it to tracking web browsers. Now, what do I mean by tracking web browsers? Two things: one is, if you go to a website on day A, and then you come back 3 weeks later, and you do something else, can the website link those two acts of yours together? And also, if on a given day you go to two different websites, and you maybe look up one fact on one, and then another fact on another, then the question is: could these two sites get together and combine the facts and get a deeper picture about you? Either of those would be tracking.
Now, there are at least three ways that are widely discussed to track web browsers. You can use cookies, which are these little bits of information your computer will store and send back, and sites can put serial numbers and tracking numbers in them. But of course we all know about cookies. You all have browser settings to delete them or limit them. So we can survive cookie tracking.
– Cookies
– IP addresses
– Supercookies
You can think of these things as hoops that you have to jump through in order to get privacy on the web. If you wanna not be tracked, you have to avoid tracking by cookies, avoid tracking by IP addresses, avoid tracking by supercookies. And then if you do that, it’s time to talk about whether you can be tracked by a fingerprint.
So, a fingerprint is like the example of tracking someone with some facts like the ZIP code and a date of birth. It turns out that the characteristics that the web browsers have, like the version of the browser, what operating system it’s on, etc., combine together in the same way as those other facts and perhaps they make your browser unique.
Now, there are different degrees of uniqueness that you might get out of the version information of your browser, you might have complete global uniqueness. So you sitting there in the 3rd row, your browser is completely unique in the whole wide world, and we know it. When we see it, we can track your browser. But perhaps that’s not true. Even so, browser fingerprints may be a problem because they mean that your IP address combined with your fingerprint is uniquely identifying.
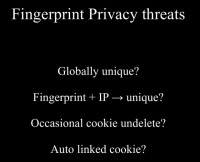
And the last thing that makes fingerprints really nasty is that unlike cookies, they are automatically the same when different sites collect them. So if a website over here sees your fingerprint and a website over there sees your fingerprint – they see the same thing. If they both tracked you with cookies they’d get different cookies, and they’d have to have some sneaky process to link them together, whereas fingerprints are automatically the same.
We heard a lot of rumors at EFF about fingerprints. We heard that some web tracking and analytics companies have started using them. We heard rumors that web based DRM3 systems were using fingerprints to track people. We heard that they were being used as a backup authentication mechanism for financial systems, which is maybe less of a problem than first two.
And we were really curious about how affective this method was. We also worried about a more mundane question, which is every single time you go to a web site, almost all websites are configured to log your browser’s User-Agent string. And we were wondering how much of a problem that was, so we decided to get some numbers to find out.
We put up this little website – www.panopticlick.eff.org. And if you went to that website – you can still go there – you’d see a page that tells you what’s going on and then gives you a little button you can click if you want to be part of the experiment. And if you click on that you get a page that says: “Oh, your browser appears to be unique, conveys up to 20 bits, possibly more, of identifying information coming from your fingerprint”. And then there is this little table showing what all the component measurements were, and how identifying each of those works.
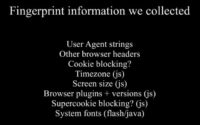
It will turn out that the 2 most problematic ones – they are all kind of problematic – but the 2 most problematic ones of these plugins here are these fonts (Flash/Java) at the bottom.
So there are a lot of things we didn’t collect but which you could use to make these fingerprints even nastier. And in fact, it turns out we’ve seen some companies in the private sector that will sell you a fingerprinting system that doesn’t just do the kind of 8 things we did but actually also it does a lot of other stuff.
One particularly nasty thing is you can measure the clock skew of the quartz crystal – that is, how much faster or slower than another clock your computer is. And that’s very hard to hide and it’s unique to your hardware rather than just to your software. You can measure the characteristics of the operating system’s TCP/IP implementation. You can measure the order in which the headers show up.
There is lot of stuff in ActiveX, Silverlight and other Adobe libraries that we didn’t have time to dig through and find and use, but you can do that. There are quirks to the way that each browser has implemented JavaScript that could be identified with the right code. There is a really nasty bug that just recently started being fixed in browsers where you can measure the history of a browser using CSS detection.
And some of these things we didn’t collect because we just didn’t have time to implement them. Some we didn’t collect because we didn’t know about them. Once we put up the site, we got a lot of emails saying: “Hey, you could collect these things as well”. And lastly, some things like the CSS history, we were not sure would be stable enough to include in a fingerprint without some kind of fuzzy matching code that we didn’t have.
So, the point here is that all of our results should be taken as a kind of optimistic story about how much privacy you have: with a really good fingerprint, the fingerprint is more powerful and more revealing.
So the way we handled the data of our site is we set up a 3-month persistent cookie and we stored an encrypted IP address with a key that we threw away. And we used those primarily to avoid counting you twice. If you came back we wanted to know if you were the same person with the same fingerprint or another person with the same fingerprint, because those two are very different things.
And we had an exception for that, which is if at a particular IP address we saw one cookie A and then cookie B, and then cookie A again, we thought that’s probably, or at least potentially, evidence that there are more than two computers behind that IP address that pass behind the NAT firewall, that have the same fingerprint. And that could be important because it could be a sign that there is a corporate network there and that there is some sysadmin who clones all the same code out to all the machines everyday. And so there are genuinely multiple machines with identical fingerprints there, and that gives the people in that office some protection. So we decided not to treat those as repeat visits from the same browser if they were interleaved.
One thing to note is a lot of people are confused by the numbers we have on our site. Those use the cookies but not the other methods of avoiding double counting, because we had to compute that stuff on the fly for millions of visitors. The data set I am presenting in this talk has more fancy control for that stuff.
So we got a pretty big data set. We had 2 million hits, about a million distinct browser instances there. The people that we were measuring were not representative of the entire web user base. People who come to EFF site are mostly people who know about and care about privacy. However, as I said before, you have to jump through three hoops to not be tractable on the web. So we think this is kind of a relevant data set to ask. The people who block cookies and know about IP addresses and how to hide them and so forth – end up being tractable by their fingerprints instead. And another point is that data in this talk is all based on the first 470,000 instances – the first half of the data set.
83.6% had completely unique fingerprints
(entropy: 18.1 bits, or more)
94.2% of “typical desktop browsers” were unique
(entropy: 18.8 bits, or more)
It turned out people were really unique. 84% of the browsers that came to our test site were unique completely in the data set. If you split the data set up, and say let’s just look at the browsers that have either Flash or Java installed – and that’s the best relevance set if you are talking about desktop browsers – your uniqueness rates go up to 94%. And only 1% have a fingerprint that we saw more than twice.
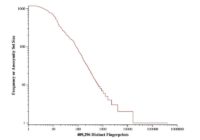
There is an interesting statistical question you might ask, which is, okay, sure you saw 94% or 84% uniqueness in your data set, but that was only 500,000 people. Would people be less unique if could get data for the whole 1 to 2 billion people who use the web? And this is an interesting statistical question. I have a theory about how to solve it involving Monte Carlo simulations1: you try a hypothesis probability distribution, you run it through a simulation, you see if it produces a graph that looks like the one I showed.
But we didn’t try to do this because in a sense our data set, which is just a measurement of privacy, is not meaningfully representative of 1 to 2 billion browsers in existence. So if someone else has a less biased data set, you could do this statistical question. We didn’t try.
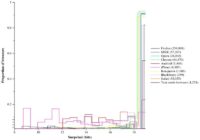
And so, if you look at this graph, anything that’s on the right-hand axis is a proportion of uniqueness. These things were completely unique in our data set. On the other end, we have the least revealing fingerprints. So let’s take an example, Firefox is the black line. It follows the curve at the bottom left, it has a little bit of a tail in the non-unique area. That’s because some people had JavaScript turned off in Firefox, or they were running Torbutton2 and it shows up as Firefox. And then, at the top right there is a very large number of unique Firefoxes.
All of the desktop browsers aside from Firefox are like that, but without the little tail of non-unique people. So generally desktop browsers are bad. One of the browsers that did well is iPhone. The iPhone does very well. It’s not very fingerprintable, it’s this purple line, and there are quite a lot of iPhones that are not unique. That’s perhaps not surprising because there aren’t yet plugins and font variation on iPhones. Really, all you are talking about is what time zone you’re in, what language you have and maybe which version of the iPhone OS you have. But there’s not very much to fingerprint iPhone with.
Android does almost as well, not quite as well because there are more iPhones than Androids, but those phone browsers look pretty good. In practice of course, they have really bad cookie settings, so people who use them probably get tracked by the cookies. But this was a good result for the phones.
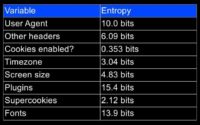
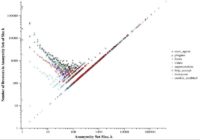
Anonymity set size of 1 means you are completely unique because of your fingerprints or your font, or your plugins. So you see up here (in the top left section) there are a lot of people who are unique: 200,000 – 250,000 people who are unique just because of the plugins they have installed on their browsers. There were 200,000 (see vertical axis from the top down) who were unique just because of their fonts. 25,000 were unique just because of their User Agent etc.
As you go from left to right, these are less identifying values. And then in the top right part of the graph, we see that having cookies enabled wasn’t a very revealing fact.
Another really interesting question you might have is: “Sure, you can identify people, but don’t these fingerprints change over time? Are they a stable way to track someone if they could upgrade their browser or install a new font and certainly the fingerprint would be different?” And so we decided to check this.
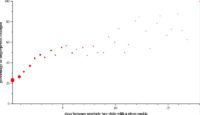
And then we said, as the function of how much later they came back, what was the probability that their fingerprint had changed? And you can see as more time has passed, the likelihood that the fingerprint was different when they came back goes up. We measured this with cookie, so there’s a cookie that you could reliably use to see the same person, and then you can see if the fingerprint changes.
So actually, fingerprints don’t last very long, the half-life of these things is 4 – 5 days. So perhaps that’s actually really good time. Perhaps fingerprints, while they are instantaneously identifying, aren’t a stable way to track people over time. Unfortunately this turned out not to be true. So the way we did that is we said: “Okay, your fingerprint has changed, can we do some kind of fuzzy matching algorithm that will see if your fingerprint later, after the change, was uniquely tieable to your fingerprint beforehand?”
And I implemented a really hacky algorithm to do this. It just says: if only 1 of those 8 measurements has changed, and it hasn’t changed very much, and that maps to a unique fingerprint from beforehand, then let’s guess that it’s you. And it only tried to do this if you had something quite revealing like Flash or Java installed. So this algorithm guesses about two thirds of the time. But when it does guess – it’s 99% accurate, so it has a 99% chance of correctly guessing which fingerprint you changed from, and less than 1% chance of getting it wrong.
Matching algorithm:
guessed 2/3 of the time
(99.1% correct; 0.9% false-positive)
So even though fingerprints change quite fast, if really the half-life of a fingerprint is 5 days, actually you are still trackable once your fingerprint has changed.
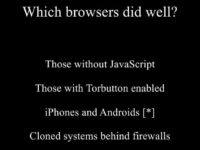
If you use Torbutton, it zaps the plugin list. The Torbutton developers knew about a lot of these attacks and anticipated them in various ways, so Torbutton – you haven’t use Tor, you can just use the little Torbutton Firefox extension, and you are in pretty good shape.
If you use an iPhone or an Android and you manage the cookie problem – you are in pretty good shape.
And lastly, you know, this small percentage of systems that were behind firewalls and appeared to have the same fingerprints: we saw about 3% of IP addresses that had multiple visitors coming from them exhibiting that kind of behavior. So that 3% of systems maybe has some kind of anonymity, although it’s a bit hard to distinguish that from the browser’s private browsing mode. And it would also be the case that if you implemented the clock skew hardware based fingerprinting, you could probably tell people apart even if they have a firewall and a clone fingerprint.
So currently there aren’t very many web browsers that do well. We also saw some other really interesting things. One interesting thing was that sometimes privacy enhancing technologies are the opposite. Something that’s designed to hide your identity turns out to be the unique thing that tracks you. If you install a Flash blocker for instance, that has a unique signature that you can tell, okay, this browser has Flash installed but we’re not gonna get an answer back from the Flash plugin when we ask it for fonts. So people who have done that were all pretty much unique.
The noteworthy exceptions to this problematic rule about privacy enhancing stuff to feeding your privacy were NoScript and Torbutton which both are fingerprintable, but the amount you gain from having them turned on outweighs the amount you lose from them.
Another lesson here is that if you are designing an API1 that’s gonna run inside the browser, you should never ever offer some call that returns a gigantic list of system information about the machine you are running on. So this was true both for the plugin list where you just ask navigator for plugins and you get back a list of all of them, and the version numbers of all of them. That’s gonna make a lot of people unique.
Similarly, don’t return the list of all the fonts. If you really need to show people a particular font, make them ask about the specific font rather than being able to ask about all fonts at once. Perhaps an even better solution to this would be to not have your system fonts display in your browser at all. Perhaps if a website wants to render some rare font like ‘Frankenstein’, it should have to give you the TTF file along with the website.
The problem here is that even if we block the bits of Java and Flash that give font lists back, there are some nasty websites out there showing that you can detect fonts using CSS which is almost unblockable. You just render the font inside an invisible box and then measure how wide it is, so if the user has the font installed the box will be width A, and if they don’t have it installed it will be width B. And there is a little cute website called flippingtypical.com that demonstrates this. So this stuff is hard to block.
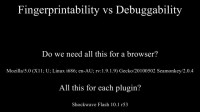
And at least, perhaps when you enter private browsing mode in your browser, it should be making that trade-off the other way around. The same is true for plugin list. Right now, if you look in the plugin list and say what version of Flash do you have, it’s not Flash or Flash 10, it’s Flash 10.1 r53. And so all these little facts, you know, you have 10 plugins and you get this version information about all of them – it adds up.
Right back at the start I said there were 4 kinds of attacks you could use fingerprinting for. One is global uniqueness, and in a lot of cases it looks like browsers are globally unique, not all cases but a lot of them. The second case where you have an IP address plus a fingerprint – then you are almost guaranteed to be able to track someone. And you can definitely undelete cookies that people have deleted, and you can definitely link these things across websites.
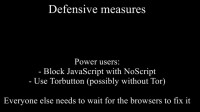
So everyone else is gonna need to wait for the browsers to find a solution to this. Fortunately they’ve sort of started. We have been talking to the Mozilla people and they were interested in attempting to fix some of the stuff, at least in private browsing mode. Google is maybe a step behind but also there’s someone interested in trying to tackle this. So perhaps we can come back in a year or two and say we’ve made a small amount of progress on the problem.